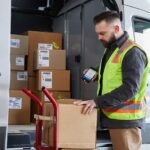
MIT student develops obstacle-detection system for drones
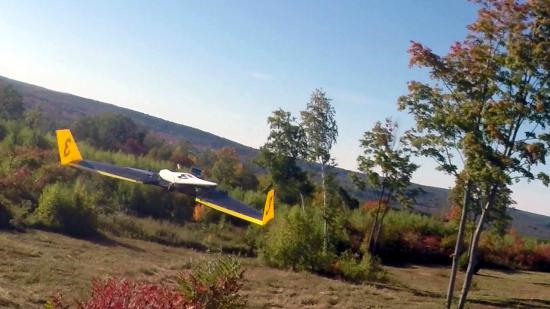
A researcher from Massachusetts Institute of Technology’s (MIT) Computer Science and Artificial Intelligence Lab (CSAIL) has developed a new obstacle-detection system for drones. According to a notice posted on CSAIL’s website, the system will allow a drone to “autonomously dip, dart and dive through a tree-filled field at upwards of 30 miles per hour”.
Andrew Barry, the CSAIL PhD student who developed the system as part of his thesis with MIT professor Russ Tedrake, was quoted as saying: “Everyone is building drones these days, but nobody knows how to get them to stop running into things.
“Sensors like lidar are too heavy to put on small aircraft, and creating maps of the environment in advance isn’t practical. If we want drones that can fly quickly and navigate in the real world, we need better, faster algorithms.”
Essentially, Barry realised that the algorithms could be speeded up if you reduced the amount of information that the system has to process. Traditionally, drones create their view of the world by using images captured on cameras and search through the depth-field at multiple distances – one metre, two metres, three metres, etc. – to determine if an object is in the drone’s path.
However, according to the CSAIL notice, such approaches “are computationally intensive, meaning that the drone cannot fly any faster than five or six miles per hour without specialized processing hardware”.
The CSAIL notice continued: “Barry’s realization was that, at the fast speeds that his drone could travel, the world simply does not change much between frames. Because of that, he could get away with computing just a small subset of measurements – specifically, distances of 10 metres away.”
According to Barry: ““You don’t have to know about anything that’s closer or further than that. As you fly, you push that 10-metre horizon forward, and, as long as your first 10 metres are clear, you can build a full map of the world around you.”
The software recovers the missing depth information by integrating results from the drone’s odometry and previous distances.