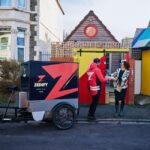
The realistic future for AI in last-mile tech
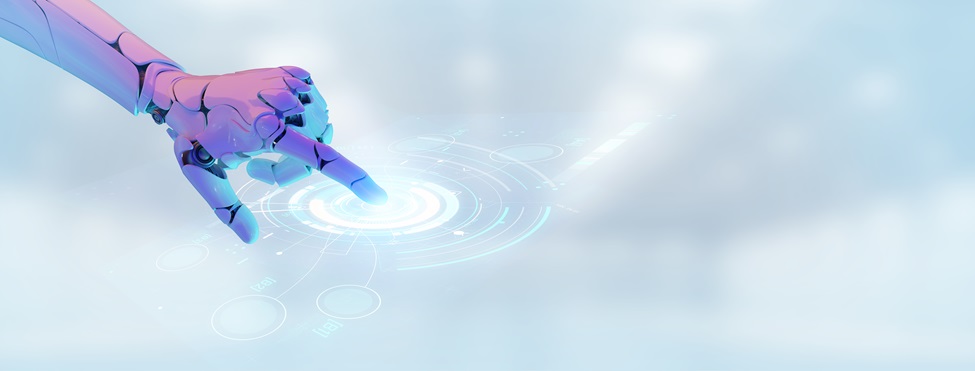
2023 will be remembered as the year where Artificial intelligence (AI) went massively mainstream, with chatbot interfaces powered by Large Language Models (LLMs) such as ChatGPT capturing the public imagination and attracting massive attention in the world of business. Doddle’s Chief Strategy Officer Bob Griffiths, discusses the potential impact of AI on the sector.
“The hype is extraordinary. In June, a one-month-old AI startup, Mistral, raised €104 million to compete with the likes of OpenAI, ChatGPT’s creator, which itself has been heavily funded by Microsoft.
The next questions have been in everyone’s minds (and on their newsfeeds) for months at this point. What can such a technology change? What will AI do to our industries? At a micro level, will our jobs significantly change, and at a macro level, will the entire nature of last-mile logistics have to change too?
Well, to put it bluntly, Large Language Models (the buzziest and most commonly-discussed form of AI) are going to have a fairly minor impact on the physical realities of ecommerce logistics. The ability to generate text or even images in response to user prompts is simply not all that relevant to the needs of consumers, retailers or logistics providers in efficiently and effectively delivering and returning goods.
That’s not to say that AI won’t massively reshape the world. It will just take time to do that. That said, much of the basis for that change is already here with us today. Alexa and Siri are reasonably powerful AI tools that have been in our homes for years. What’s changing is that AI is now evolving faster than ever.
The type of AI that could actually truly change the future of last-mile logistics will still have to come about incrementally, through the slow accumulation of intelligence added to different elements of each package’s journey, and the deeper knitting together of each of those elements into an end-to-end intelligent platform.
It might be a long way off – but some of the elements are already extant in today’s technology solutions. Let’s look at where we are already, and chart a path from there to the pretty amazing potential future that an artificially intelligent last-mile logistics system could represent.
Intelligence in today’s delivery and returns operations
Technologically, the biggest difference between the kinds of rules-based, specific solutions we see in the market today and something that would be worthy of the term ‘artificial intelligence’ is that rules-based systems are configured by people. They consider a limited number of inputs, setting up rules for each, with the aim of achieving specific goals. An AI in the same area would be able to take thousands more inputs into account, using a sophisticated machine learning model, and predict the outcomes of its actions. It requires lots of training and data to shape the model, but then it operates at a scale far beyond a rules engine, and with predictive and analytical capabilities that a rules engine model cannot offer.
Let’s take the example of returns. Today, rules-based intelligence enables businesses like Amazon and retailers using Doddle’s Digital Returns platform to treat individual returns differently depending on a list of characteristics. Those rules are set by the retailer to try to drive a balance of efficiency and customer loyalty.
The best digital returns solutions can intelligently adapt the options (and costs) presented to consumers based on order information, item details, dimensions, category or previous returns behaviour. The processing can be similarly configured to treat items differently depending on similar data. The returns journey can be customised based on pre-configured rules, effectively basic, user-created algorithms. That means retailers can direct returns to different locations, for example, depending on a variety of factors – something that wouldn’t be possible with a pre-printed shipping label.
Amazon is the market leader in this type of ‘rules engine’, from both the retail and carrier perspectives. Customers initiate a return from their order list, and the options available to them (and the pricing of those options) are determined by various factors, including their return reason, the type of item being returned, the cost to Amazon of the return, the availability of local Amazon-owned drop-off points, et cetera. This means that a damaged item, or an item that was delivered late, are usually eligible for completely free return shipping and refund. However, ‘buyer’s remorse’ returns are usually charged, and the charge varies depending on the drop-off method.
It’s not just Amazon that has access to the technology though. Logistics businesses like Australia Post are able to provide rules-based intelligent returns platforms to their customers, powered by Doddle’s Digital Returns solution, automating decision-making in returns to maximise revenue recovery and reduce processing costs.
However, most rules-based solutions can only account for a relatively short list of available inputs. For most carriers, even if they do offer a digital returns solution, something like pricing is usually a fixed element. Pricing a return differently depending on return reason, or estimated processing cost, is very rare. It’s even rarer for the customer insights generated in an intelligent returns process to be integrated with other tools or systems, like a customer relationship management (CRM) platform for example. That means the carrier and retailer might not be able to extract and utilise the insights derived from the huge quantity of data that such a returns solution could capture.
As an example, even the extremely digitally-enabled ASOS admitted that 6% of its customer base managed to constitute a loss of over £100 million, thanks to their high order volume and significant return rate (and low basket value). What went unsaid in ASOS’ reporting is whether or not those customers were still incentivised to come back and shop more with discounts and other promotional activity – did the ASOS marketing team have the data to filter out from their campaigns those customers who actively cost the business money through their returns profile, or was it still courting them based on the fact that they were high-frequency, engaged shoppers?
These examples illustrate both the potential and the current limitations of rules engines. While they can allow a significant degree of personalisation, and account for lots of important factors, it’s simply very difficult to manually calculate out what the best outcome will be when more and more inputs are involved, and it’s also hard to integrate more of those inputs into a rules engine. That’s true within businesses (integrating between systems and departments) and between businesses (for example, a parcel carrier integrating with a retailer to utilise customer data to offer a personalised set of delivery or returns options).
Even where machine learning and artificial intelligence does currently exist in logistics tech, it’s often isolated from other useful data. Neural networks have been used in determining the most efficient routing for parcel deliveries for years now. The routing algorithms which dictate exactly how couriers should drive from drop to drop are an example of the important but still limited ways in which artificial intelligence is currently deployed in logistics: in a single area, improving efficiency but not interacting with any other source of data. For example, many don’t bring in data around carding (also known as failed deliveries) and what time of day deliveries are most likely to succeed, which might be a way to improve the overall effectiveness of a delivery route.
The AI Future
In the future of the last mile, one of the potential uses of artificial intelligence is to create true personalisation – ensuring customers are offered the right delivery and returns options at the right price, throughout their journey, depending on the myriad and customer-specific factors that impact the cost and experience of delivery and returns.
That could look like a checkout tool offered by a parcel carrier to their merchant customers, which improves conversion for retailers by recommending the best delivery option for each individual customer. It could draw on existing address data to match delivery success rate against the forecasted time of delivery. From its data sources, the model might determine that while home delivery is 99% likely to succeed for one customer on a Wednesday afternoon, another only has a 55% chance of being available to take delivery at that time, and they live in an apartment where leave-safe isn’t a good option either. In the first case, the model would recommend home delivery, in the latter it might suggest that the customer should use a nearby parcel locker to ensure they get their parcel as fast and securely as possible.
That’s one fairly simple example, but the point of introducing machine learning as opposed to human-configured rules is that such a tool could theoretically account for hundreds of data points when choosing which delivery options to recommend to a customer. That makes it hugely powerful and scalable, at the cost of requiring massive sources of data to make it a better alternative to a simple rules-based system.
However, the biggest logistics businesses in the world certainly already have the data and the scale to make such efforts viable. FedEx has spun up its FedEx Dataworks division to harness the data from its 17 million daily global shipments, which it enriches with traffic and weather information to predict the likely success of a delivery. That’s currently used for high-priority deliveries, but scaling such a solution across a whole network should also have massive benefits – if FedEx could reduce its failed delivery rate worldwide by 1%, tens of thousands of extra parcels get delivered every day.
FedEx is taking the most important step towards AI becoming viable – investing time and resource in testing and learning. The technology is developed enough to support logistics changing for the better. Now it’s incumbent upon logistics businesses to prioritise it and start developing their experience with AI, so that these small steps can become giant leaps over time.”
About Bob
Bob has led the product team at Doddle since 2016, focusing on helping carriers and posts to benefit from cutting-edge technologies in the first and last mile.